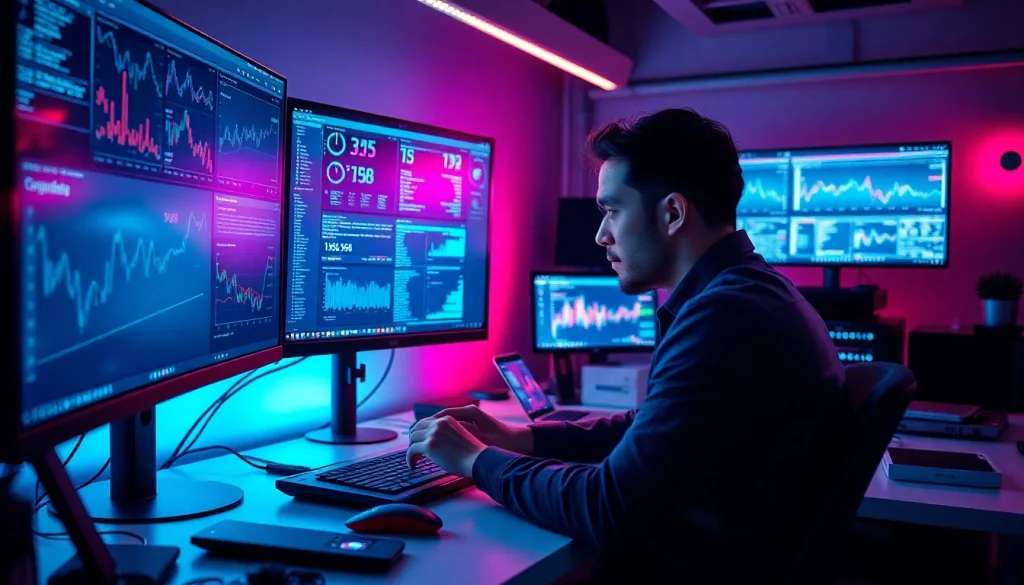
The Evolution of Data Analytics
Data analytics has undergone a significant transformation from its inception to the present day. Once limited to basic statistical analysis, it has evolved into a sophisticated field where organizations leverage vast amounts of data to drive decision-making and gain a competitive edge. The rise of big data, artificial intelligence, and machine learning has further propelled the advancement of data analytics, making it an indispensable tool in today’s business environment. Understanding the evolution of data analytics can help professionals appreciate its potential and integrate effective strategies into their operations. Organizations like www.informaticsview.com are at the forefront of this evolution, offering robust analytics solutions that optimize business processes.
Understanding the Basics of Data Analytics
At its core, data analytics involves the systematic computational analysis of data to inform business decisions. This foundational understanding involves breaking down data into various categories, such as descriptive, diagnostic, predictive, and prescriptive analytics. Each category serves a unique purpose:
- Descriptive Analytics: This type focuses on summarizing historical data to understand trends and patterns.
- Diagnostic Analytics: It explores data to answer why something happened by identifying correlations and factors leading to past outcomes.
- Predictive Analytics: This involves using historical data to forecast future events, employing statistical models and machine learning techniques.
- Prescriptive Analytics: This advanced form offers actionable recommendations based on data analysis, helping organizations make informed decisions.
These categories are not mutually exclusive; together, they form a comprehensive analytics framework that organizations can utilize to gauge performance, streamline operations, and anticipate future challenges.
Key Tools and Technologies in Data Analytics
The landscape of data analytics is rich with tools and technologies that facilitate the collection, processing, and visualization of data. Some key tools include:
- Data Visualization Tools: Tools like Tableau, Power BI, and Google Data Studio help transform data into comprehensible visual formats, making it easier to interpret and communicate insights.
- Cloud Storage Solutions: Platforms like Amazon Web Services (AWS) and Microsoft Azure offer scalable solutions for storing enormous data volumes, enabling organizations to access data from anywhere.
- Statistical Software: R and Python libraries provide powerful options for performing statistical analysis and developing machine learning models.
- Business Intelligence Platforms: These platforms aggregate data from various sources and provide dashboards and reports to aid in strategic decision-making.
As the technology evolves, staying abreast of the latest tools and practices is vital for data professionals looking to maximize their effectiveness in data analytics.
The Role of Data in Modern Business Strategies
In modern business landscapes, the role of data cannot be overstated. Companies are increasingly recognizing the need to harness data insights for competitive advantage. Effective data transformation leads to improved customer experiences, operational efficiencies, and innovative product development. Here’s how data influences business strategies:
- Data-Driven Decision Making: Organizations utilize data insights to guide their strategic direction, ensuring that decisions are based on empirical evidence rather than intuition alone.
- Personalization: Data analytics empowers businesses to tailor their products and marketing strategies to meet individual customer preferences, enhancing customer loyalty and engagement.
- Operational Efficiency: By analyzing workflow processes and identifying bottlenecks, companies can optimize operations, reducing costs and improving service delivery.
- Innovation: Insights derived from data analysis can inform product development, guiding firms in creating solutions that meet emerging consumer needs.
As businesses continue to pivot towards data-centric models, those with robust data analytics frameworks will be better positioned to navigate challenges and seize opportunities.
Key Features of www.informaticsview.com
User-Friendly Interface for Data Exploration
One of the standout features of www.informaticsview.com is its user-friendly interface. Designed with the end user in mind, it enables users—regardless of their technical expertise—to explore data intuitively. The dashboard simplifies navigation through various data sets and analytical tools, allowing users to access insights quickly without a steep learning curve. This ease of use encourages widespread adoption within organizations, empowering teams to leverage data for day-to-day decisions seamlessly.
Integration Capabilities with Other Platforms
Integration is crucial in today’s multi-platform business environment. The capabilities offered by www.informaticsview.com allow businesses to connect their existing data infrastructure with other essential tools and systems, such as CRM platforms, financial software, and marketing technologies. This seamless data flow ensures that businesses maintain a single source of truth and eliminate silos, promoting a holistic view of organizational performance.
Customizable Data Reporting Options
Reporting is a vital aspect of analytics, and the customizable reporting features of www.informaticsview.com serve to enhance this function significantly. Users can tailor reports to focus on the most relevant metrics and KPIs, ensuring that stakeholders receive insights that matter most to them. This customization promotes clarity and drives actionable insights, making it easier for decision-makers to understand performance and adjust strategies accordingly.
Best Practices for Effective Data Analysis
Identifying Relevant Data Metrics
Effective data analysis requires identifying the right metrics to track. Organizations should begin by establishing clear objectives for their data analysis initiatives. Once goals are defined, selecting metrics that align with these objectives is critical. Relevant metrics could include:
- Sales Growth Rate: A measure of revenue increase over a specific period, indicating market response.
- Customer Acquisition Cost (CAC): This metric evaluates the cost-effectiveness of gaining new customers, helping to inform marketing strategies.
- Customer Lifetime Value (CLV): Understanding the long-term value a customer brings assists businesses in optimizing their customer engagement efforts.
- Net Promoter Score (NPS): This metric gauges customer satisfaction and loyalty by assessing the likelihood of customers recommending your service or product.
By meticulously selecting these metrics, organizations can ensure their data analysis is relevant and achieves its intended outcomes.
Utilizing Visualization Techniques
Data visualization is a powerful tool that enhances understanding through graphical representation. Effective visualization techniques can reveal patterns and insights that might not be apparent in raw data forms. Common techniques include:
- Bar Charts and Histograms: These facilitate comparison among categories and display distribution within data sets.
- Line Graphs: Ideal for showcasing trends over time, helping organizations to visualize changes and forecast future developments.
- Pie Charts: Useful for illustrating proportional relationships, such as market share distribution among competitors.
- Heat Maps: These accentuate areas of high and low activity within a data set, useful for spatial data analysis.
Incorporating these visualization techniques into reporting practices not only enhances communication with stakeholders but also supports more informed decision-making.
Implementing Continuous Improvement Processes
Data analysis should not be a one-off endeavor; instead, it should uphold a principle of continuous improvement. Organizations can adopt methodologies like Six Sigma or Lean to instill iterative processes that assess performance regularly. By analyzing data consistently and seeking areas for enhancement, companies can foster an environment of progress. Workflow audits, customer feedback loops, and training programs can all contribute to this ongoing improvement process. Ultimately, this commitment to evolution allows businesses to adapt to changing markets and consumer demands effectively.
Challenges in Data Analysis and Solutions
Common Pitfalls in Data Interpretation
Data interpretation can be fraught with challenges, leading to potential pitfalls that can distort decision-making processes. Common issues include:
- Confirmation Bias: Analysts may inadvertently favor data that supports preconceived notions while disregarding contradictory evidence, skewing results.
- Overlooking Context: Numbers alone can be misleading without context. Analysts must consider external factors influencing data trends.
- Reliance on Outdated Data: Decision-making based on obsolete information can lead to misguided strategies. Regular updates and real-time analytics are essential.
To overcome these pitfalls, organizations should cultivate a culture of critical thinking, solicit diverse perspectives, and leverage advanced analytics tools to catch potential biases or data inaccuracies early on.
Strategies to Overcome Data Overload
In an age of big data, organizations often face the challenge of data overload, where excessive information makes it difficult to discern valuable insights. Strategies to mitigate this issue include:
- Data Prioritization: Establish criteria for prioritizing data based on relevance to business objectives and the insights they can yield.
- Automated Analytics Tools: Leverage artificial intelligence and machine learning tools that can process vast quantities of data efficiently, highlighting essential insights.
- Clear Data Governance: Implementing policies that define data access, usage, and management can help streamline data processes and maintain focus on what truly matters.
By employing these strategies, companies can focus on quality rather than quantity, thereby maximizing the effectiveness of their data analysis activities.
Ensuring Data Accuracy and Integrity
Data accuracy and integrity are paramount for effective analytics. Inaccurate data can lead to misguided business strategies and lost opportunities. To ensure data quality, organizations should take proactive steps, such as:
- Regular Audits: Conduct periodic reviews of data sources to check for inconsistencies, errors, and gaps that could impact analysis.
- Standardizing Data Entry: Implementing standardized protocols for data entry can reduce discrepancies and enhance data reliability.
- Training Staff: Invest in training programs to educate team members on the importance of data integrity and proper data handling practices.
By prioritizing data accuracy, organizations empower their decision-making processes and build a trustworthy foundation for their analytics practices.
Measuring Success with www.informaticsview.com
Key Performance Indicators for Data Analytics
To gauge the effectiveness of analytics efforts, organizations must establish and monitor key performance indicators (KPIs). These metrics must align with strategic goals and provide actionable insights. Here are some integral KPIs:
- Data Accuracy Rate: Measures the percentage of data entries correct and free from errors.
- Adoption Rate of Analytics Tools: Assesses how effectively teams are utilizing analytics tools within the organization.
- Return on Investment (ROI): Evaluates the financial returns from analytics initiatives compared to their costs.
- Time to Insight: Measures the time it takes to derive actionable insights from data, reflecting operational efficiency.
Establishing these KPIs allows organizations to track their analytics maturity, identify areas for improvement, and gauge the overall impact on business performance.
Case Studies of Successful Implementations
Real-world case studies can provide compelling evidence of how effective data analytics can transform organizations. Consider the following examples:
- Retail Industry: A major retail chain utilized predictive analytics to optimize inventory management, resulting in a 15% reduction in excess stock and a 10% increase in sales due to improved product availability.
- Healthcare Sector: A healthcare provider implemented data analytics to analyze patient outcomes and streamline processes. This led to a 20% decrease in patient readmission rates, demonstrating the potential of data-driven insights in improving patient care.
- Financial Services: A bank leveraged customer segmentation analysis to develop targeted marketing strategies, subsequently increasing new account openings by 30% within six months.
Such case studies highlight the tangible benefits that organizations can achieve through effective data analytics practices.
Future Trends in Data Analytics Performance Metrics
The field of data analytics is continually evolving, and staying ahead of the trends is essential for organizations. Here are some predicted trends in performance metrics:
- Real-Time Analytics: The demand for real-time insights will increase as businesses strive for agility, necessitating tools that can provide instantaneous data analysis.
- AI-Powered Analytics: The integration of artificial intelligence in analytics will allow organizations to automate data processes and generate deeper insights more efficiently.
- Enhanced Data Stewardship: Organizations will place greater emphasis on data governance practices to ensure compliance with regulations and build consumer trust.
By anticipating these trends, organizations can adjust their analytics strategies proactively, ensuring they remain competitive in a data-driven world.