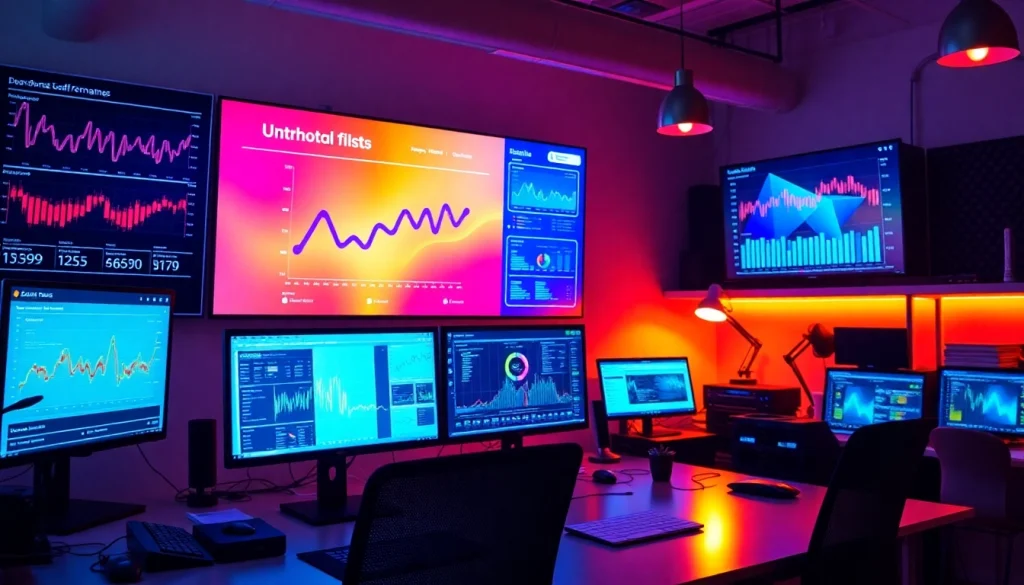
Understanding the Basics of Data Analytics
In the modern business landscape, data has become one of the most valuable assets for organizations across various sectors. Understanding data analytics is essential for leveraging this asset effectively. Analytics transforms raw data into meaningful insights, aiding in decision-making and strategic planning. From identifying trends to predicting future outcomes, the role of data analytics cannot be overstated. This is the foundation on which successful enterprises build their competitive edge. For more insights on effective analytics strategies, visit www.informaticsview.com.
What is Data Analytics?
Data analytics refers to the process of examining, cleaning, transforming, and modeling data with the goal of discovering useful information, informing conclusions, and supporting decision-making. It involves tools and techniques from various fields such as statistics, computer science, and machine learning. By employing algorithms and statistical methods, organizations can analyze vast amounts of data to extract valuable insights that drive business decisions.
Importance of Data in Decision Making
Informed decision-making is crucial for any organization aiming to stay competitive. Data analytics enhances decision-making processes by providing empirical evidence to back strategic choices. Organizations that leverage data effectively can respond to market changes with agility, tailor products to meet customer needs, and optimize operational efficiency. Data-driven decisions minimize risks and increase the likelihood of success in various initiatives.
Key Terms and Concepts in Data Analytics
Understanding the terminology is vital for anyone looking to delve into data analytics. Key terms include:
- Big Data: Refers to extremely large datasets that may be analyzed computationally to reveal patterns, trends, and associations.
- Machine Learning: A branch of artificial intelligence that focuses on the development of algorithms that enable computers to learn from and make predictions based on data.
- Data Mining: The practice of examining large pre-existing databases to generate new information.
- Predictive Analytics: Techniques that use statistical models and machine learning to analyze historical data and predict future events.
Essential Tools and Technologies for Data Analytics
Introduction to Data Analytics Tools
Today, a plethora of tools and technologies are available to facilitate data analytics. These tools provide a range of functionalities from data fetching and cleaning to advanced analytics and visualization. Selecting the right tools can significantly enhance an organization’s analytical capabilities.
Comparing Top Data Analytics Platforms
Several data analytics platforms offer various features. Some of the leading platforms include:
- Tableau: Known for its visualization capabilities, Tableau helps users understand their data through interactive, shareable dashboards.
- Power BI: A powerful business analytics tool from Microsoft that provides interactive visualizations and business intelligence capabilities.
- SAS: Offers a robust suite of analytics tools that allow for advanced statistical analysis and data management.
- Google Analytics: A web analytics service providing detailed statistics and insights about website traffic and user behavior.
Choosing the Right Tools for Your Needs
Selecting the right data analytics tools hinges on your specific needs and the nature of your data. Consider factors such as scalability, ease of use, integration capabilities with existing systems, and the types of analyses you wish to conduct. Organizations should also evaluate the analytics maturity of their teams to ensure that the tools chosen are accessible and effectively utilized.
Implementing Data Analytics in Your Organization
Steps to Integrate Data Analytics
Integrating data analytics into an organization requires a structured approach. Key steps include:
- Define Objectives: Clearly outline what you hope to achieve with analytics. This could range from improving customer satisfaction to enhancing operational efficiency.
- Data Collection: Gather relevant data from internal and external sources. Ensure that the data is clean and accurate.
- Choose Analytics Tools: As discussed, select tools that align with your goals and team capabilities.
- Build a Data Team: Assemble a cross-functional team equipped with the necessary skills, including data scientists and analysts.
- Analyze and Interpret Data: Apply analytics techniques to generate insights from your data.
- Communicate Insights: Present findings in a format that stakeholders can understand and act upon.
- Iterate and Improve: Regularly assess the analytics processes and outcomes to refine methodologies.
Common Challenges in Data Implementation
Implementing data analytics is not without challenges. Common issues include:
- Data Quality: Poor data quality can lead to misleading insights. It is crucial to establish data governance practices to maintain high standards.
- Skill Gaps: A lack of skilled personnel can hinder effective data analysis. Investing in training and development can bridge these gaps.
- Change Resistance: Employees may resist adopting new technologies. Encouraging a data-driven culture can facilitate smoother transitions.
- Budget Constraints: Implementing robust analytics solutions can be costly. Prioritize key projects to ensure effective use of resources.
Case Studies: Successful Data Analytics Adoption
Many organizations have successfully harnessed the power of data analytics. Consider the following case studies:
- Netflix: By analyzing viewer preferences and behaviors, Netflix has developed custom recommendations that have significantly enhanced user engagement and retention.
- Amazon: Amazon uses predictive analytics to manage inventory and streamline its supply chain, ensuring that products are available where and when they are needed.
- Starbucks: Starbucks leverages data analytics to understand customer preferences and optimize their store locations, leading to increased sales and customer satisfaction.
Measuring Success and Performance Metrics
Key Performance Indicators in Data Analytics
Establishing clear performance metrics is crucial for measuring the success of analytics initiatives. Common KPIs include:
- Return on Investment (ROI): Measuring the financial return gained from analytics initiatives.
- Customer Satisfaction: Assessing improvements in customer experience resulting from data-driven decisions.
- Operational Efficiency: Evaluating how analytics has improved processes and reduced waste.
- Data Quality Metrics: Measuring the accuracy, completeness, and reliability of data being used.
Evaluating the Impact of Analytics on Business
To evaluate the impact of analytics, organizations should regularly review performance data against established goals. This review process includes qualitative feedback from employees and customers, as well as quantitative data from KPIs. Effective evaluation techniques must foster continuous improvement.
Continuous Improvement through Data Insights
Data analytics is not a one-time initiative but a continuous cycle of improvement. Organizations should continuously analyze data to identify new opportunities and challenges, applying insights to enhance products, services, and processes. Regularly asking questions like “What do the data tell us?” or “How can we apply these insights?” helps maintain a growth mindset within the organization.
The Future of Data Analytics and Emerging Trends
Upcoming Technologies in Data Analytics
The field of data analytics is constantly evolving, with new technologies emerging regularly. Technologies to watch include:
- Augmented Analytics: This uses machine learning to automate data preparation and insight generation, making analytics accessible to non-experts.
- Data Fabric: A unified data management architecture that simplifies data access and sharing across an entire organization.
- Real-time Analytics: Enabling organizations to analyze data as it is created, providing immediate insights that drive timely decision-making.
How AI is Shaping Data Analytics
Artificial intelligence (AI) plays a pivotal role in transforming data analytics. From automating routine tasks to providing advanced predictive analytics capabilities, AI enhances the depth and speed of insights. Machine learning algorithms can process vast datasets to uncover hidden patterns, enabling organizations to make sense of data quickly and efficiently.
Preparing for Trends in Data Management
As data continues to grow exponentially, organizations must prepare for future data management trends. This includes adopting cloud-based solutions, improving data governance frameworks, and enhancing data security measures. Staying proactive about these trends is crucial for organizations aiming to maintain their competitive edge in a data-driven world.