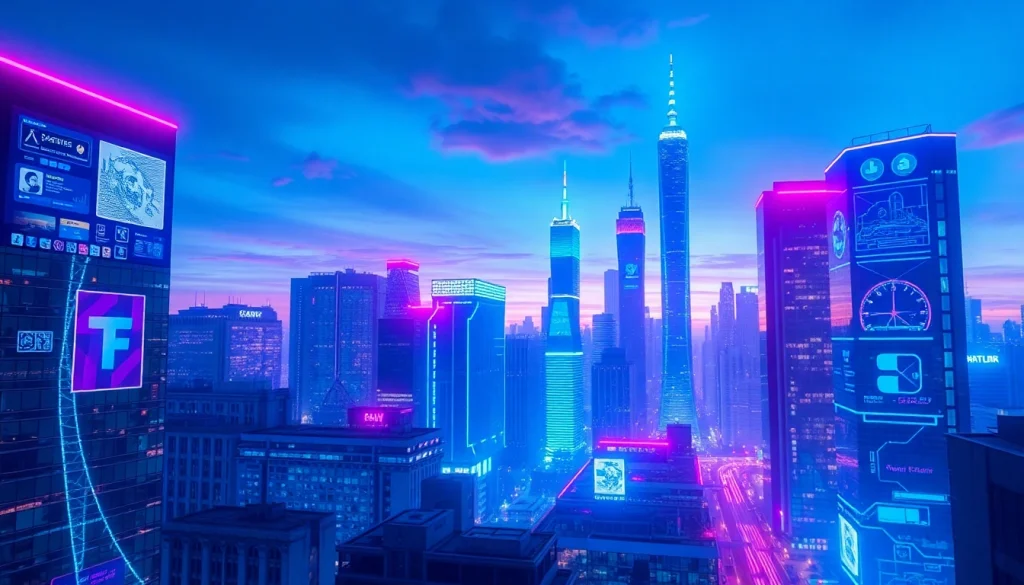
The Evolution of AI Search Technologies
The digital landscape is undergoing a seismic shift with the advent of AI search technologies. From traditional keyword-based engines to advanced machine learning algorithms, the search paradigm is transforming. In this section, we explore the historical development, key technological advancements, and how AI search stacks up against traditional search methodologies.
History and Background of AI Search
The search engine as we know it began in the mid-1990s, with the launch of pioneering platforms like AltaVista and Lycos. These engines relied heavily on keyword matching, which, while revolutionary for its time, often yielded inaccurate and irrelevant results. Fast forward to the introduction of Google in 1998, which utilized PageRank—a system that ranked web pages based on incoming links and relevance—leading to a significant improvement in search quality.
However, as information explosion accelerated, the limitations of traditional search engines became apparent. They struggled with understanding context, semantic nuances, and user intent. Enter AI search technologies: harnessing machine learning, natural language processing (NLP), and massive datasets, AI search aims to transcend the limitations of its predecessors. Not only do these systems learn from user interactions, but they can also adapt and evolve, ensuring that search results become more personalized and contextual over time.
Key Technologies Driving AI Search
AI search relies on several key technologies that work in tandem to provide superior search capabilities:
- Natural Language Processing (NLP): NLP enables machines to understand and interpret human language. By integrating NLP, AI search engines can process queries in a way that mirrors human thought patterns, offering more relevant answers.
- Machine Learning (ML): Through algorithms that learn from data, AI systems can improve over time. Whether it’s through supervised, unsupervised, or reinforcement learning, ML allows AI search engines to refine their output based on user behavior and preferences.
- Deep Learning: A subset of machine learning, deep learning employs neural networks with many layers to analyze various parameters. This technology helps AI search engines make sense of complex data sets, leading to more nuanced understanding and targeting.
- Knowledge Graphs: These structured representations of knowledge allow AI search engines to provide contextually relevant information by linking entities and concepts. Knowledge graphs are fundamental to semantic search capabilities, enhancing information retrieval.
Comparative Analysis of Traditional vs AI Search
The implementation of AI technology in search has led to a pivotal comparison between traditional and AI-driven search frameworks. Here’s how they differ:
Aspect | Traditional Search | AI Search |
---|---|---|
Query Understanding | Keyword matching, limited context understanding | Contextual comprehension, semantic analysis |
Result Relevance | Heavily reliant on rankings | Personalized results based on user intent |
Adaptability | Static algorithms | Dynamic learning from user interactions |
Data Handling | Structured data only | Unstructured and semi-structured data capabilities |
This comparative analysis reveals that AI search represents a significant leap forward. By prioritizing user-centric results and refining the search experience, AI technologies are establishing a new standard for information retrieval.
How AI Search Improves User Experience
Enhancing user experience is at the heart of AI search technologies. By embracing personalization and advanced algorithms, AI search is rethinking how users interact with information.
Personalization and Relevance in Search Results
AI search engines analyze user behavior, preferences, and past interactions to deliver personalized results. This capability is crucial today; users expect tailored content that aligns with their specific needs. Companies like Google and Microsoft are employing advanced algorithms to track user behavior. For instance, Google’s Gemini integrates user feedback to refine its search capabilities continually.
As these engines gather more data, they become adept at predicting potential user queries even before they are fully articulated. This leads to a more efficient search process, where users engage with content more likely to meet their expectations.
Speed and Efficiency of AI-Driven Searches
Another significant advantage of AI search technologies is their speed and efficiency. Traditional search engines would require longer query processing times, often resulting in frustrating wait times for users. AI search engines use advanced data processing capabilities that significantly reduce latency, enabling quick retrieval of information.
The integration of techniques such as Retrieval-Augmented Generation (RAG) exemplifies this. Systems like Azure AI Search enhance the speed of information retrieval by combining traditional search capabilities with AI-generated responses, ensuring that users receive answers swiftly while maintaining high accuracy.
Understanding User Intent with Advanced Algorithms
Understanding user intent is a pivotal factor in delivering relevant search results. AI search technologies deploy sophisticated algorithms that analyze the user’s search context, including the time of the search, location, and query history. This allows for responsive adaptations that can interpret ambiguous queries and disambiguate meanings based on situational cues.
For example, when a user searches for “jaguar,” an AI search engine can determine if they are looking for information about the animal or the car by considering their previous searches or geographic markers that indicate a local interest in cars. This intuitive grasp of intent vastly improves user satisfaction and engagement.
Implementing AI Search Solutions for Businesses
As organizations increasingly recognize the value of AI-driven search, implementing these solutions becomes imperative. Here, we discuss the steps and considerations for businesses looking to integrate AI search technologies effectively.
Assessing Your Business Needs for AI Search
Before embarking on the AI search implementation journey, businesses must first assess their specific needs. This involves understanding the nature of user queries, the volume of content, and the types of data currently in use. Organizations should evaluate:
- What types of queries are common among users?
- What data is being generated, and how is it stored?
- Are there existing gaps in information retrieval or user satisfaction?
Conducting user surveys and analyzing engagement metrics can help in identifying critical areas where AI search can make a difference. This groundwork is essential for tailoring the AI solution to meet specific business objectives.
Choosing the Right AI Search Provider
The market is replete with AI search solutions, each offering unique features, capabilities, and pricing models. For a successful implementation, businesses must consider several factors when selecting a provider:
- Scalability: As your business grows, so too will the data and user demands. Choose a provider that can scale solutions seamlessly to accommodate growth.
- Integration: Look for AI search technologies that can easily integrate with existing systems and workflows. A smooth implementation process minimizes disruption.
- Cost-Effectiveness: Ensure that the chosen solution fits within your budget while providing maximum value. Compare features across different providers to identify the best trade-offs.
- Support and Training: Adequate support and training from the provider are crucial for the successful adoption of AI search. Ensure they offer resources that facilitate learning and troubleshooting.
Case Studies: Successful Implementations of AI Search
Illustrating the real-world application of AI search technologies, several case studies provide insight into successful implementations:
- Company X: A media organization adopted an AI-powered search solution that significantly reduced content retrieval time by 70%. By leveraging machine learning to recommend content based on user behavior, they increased user engagement on their platform.
- Company Y: A retail giant integrated AI search across its e-commerce platform, resulting in personalized product recommendations. This led to a 25% increase in conversion rates as customers found relevant products faster.
- Company Z: An educational institution deployed AI search tools to help students locate resources efficiently. This implementation improved research efficiency, reducing the time spent by students searching for credible sources by nearly 50%.
The Future of AI Search in Digital Experiences
The trajectory of AI search technologies indicates exciting developments on the horizon. As digital experiences evolve, understanding emerging trends and aligning with future needs becomes critical for businesses and users alike.
Emerging Trends in AI Search Technologies
The future of AI search is marked by several emerging trends:
- Voice Search and Conversational AI: As technologies like Amazon’s Alexa and Google Assistant gain popularity, more users will engage with search through voice. AI search engines will adapt to this shift by refining their capabilities to handle conversational queries effectively.
- Multimodal Search: User preferences are changing; they want more than just text-based information. Future AI search technologies will leverage multimodal capabilities to combine text, image, and video searches for richer user experiences.
- Privacy-Focused Search: With growing concerns around data privacy, the demand for AI search solutions that prioritize user privacy and data security will increase. Providers will need to innovate while maintaining transparency in data handling practices.
The Role of AI Search in Multimodal Interactions
As user interactions evolve, AI search must also focus on multimodal experiences, integrating different content types. This includes a shift toward visual, auditory, and textual information, leading to a holistic search experience.
Consider this: If a user searches for “best camera,” an ideal AI search engine can provide a blend of images, comparison charts, user reviews, and even video demonstrations. This kind of comprehensive result enriches users’ understanding and engagement levels.
Challenges and Opportunities Ahead
Despite the exciting potential of AI search technologies, businesses will face several challenges:
- Data Quality: The effectiveness of AI search is heavily reliant on the quality of data fed into the system. Inconsistent or low-quality data can lead to poor search results.
- Technical Complexity: Implementing AI solutions requires a certain level of technical proficiency. Organizations must ensure they have the right talent or partner with capable providers.
- User Acceptance: Transitioning to AI-driven search can trigger resistance among users accustomed to traditional search experiences. Effective training and change management strategies are essential to facilitate acceptance.
On the flip side, these challenges present opportunities to enhance user experience and engagement. By leveraging AI search responsibly, organizations stand to gain significant competitive advantages, driving innovation and user satisfaction.
Best Practices for Leveraging AI Search
To make the most of AI search capabilities, businesses must adhere to best practices that harness the full potential of these technologies.
Optimizing Content for AI Search Engines
Optimization is critical for ensuring your content is discoverable and relevant in an AI-driven search environment. Key strategies include:
- Structured Data Markup: Implementing structured data helps search engines understand the context of your content, improving its visibility.
- Natural Language Optimization: Write in a way that mimics conversational speech. This tactic not only engages users better but also enhances compatibility with NLP algorithms.
- Creating Quality Content: Prioritize depth and relevance over keyword density. Quality content leads to better engagement, decreased bounce rates, and increased user trust.
Keeping Up with AI Search Trends
The field of AI search is constantly changing. Keeping abreast of new technologies, tools, and user expectations is essential. Engage in continuous learning through webinars, industry conferences, and thought leadership articles. Creating a culture of innovation within your organization will ensure you remain competitive in this dynamic environment.
Measuring Success: Key Performance Indicators
Finally, establishing and monitoring KPIs is vital for gauging the success of your AI search solutions. Key metrics include:
- User Engagement: Track how often users interact with search results and content.
- Conversion Rate: Measure the percentage of user interactions that lead to desired outcomes, such as purchases or sign-ups.
- Bounce Rate: A high bounce rate may indicate that users are not finding what they are looking for, necessitating further investigation.
- User Satisfaction: Implement surveys and feedback mechanisms to gauge user satisfaction and perception of search quality.
By meticulously applying these best practices, businesses can leverage AI search technologies to drive significant improvements in user experience and operational efficiency.